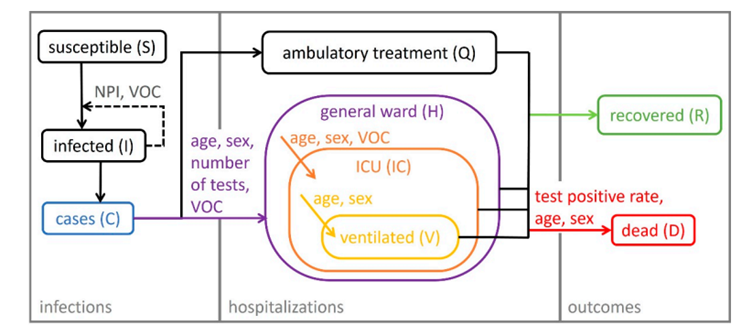
Schematic representation of the epidemiological compartment model implemented by the University of Saarland. [2, 3].
During the COVID-19 pandemic, one of the biggest challenges was the uncertainty around how well different measures – such as vaccination campaigns and lockdowns – would work. Policymakers had to make tough decisions without knowing exactly what the outcomes would be, which sometimes led to less-than-ideal results. To improve decision-making, some countries turned to mathematical models to analyse data, gain insights into the virus’s spread, the severity of the disease, the potential impact of various interventions, and to predict outcomes.
Modelling and forecasting play a critical role in public health by providing a systematic, data-driven approach to understanding disease spread and how interventions can affect it. Accurate models help anticipate potential scenarios, enabling timely and informed decision-making. This bridges the gap between theoretical planning and practical implementation, offering a data-driven foundation for public health policy. Models help identify strategies most likely to succeed under varying conditions, making them vital for preventing health crises or reducing their impact.
In Germany, the University of Saarland developed a “compartmental model” to predict how COVID-19 would spread and affect the population. This type of epidemiological model categorises the population into three disease stages: susceptible (S), infected (I), and recovered (R). It tracks how people move between these groups over time. By adjusting factors like the rate of virus transmission and the effectiveness of measures such as social distancing or vaccination, the model can simulate different scenarios and forecast how the virus might behave.
The University of Saarland’s model used data from multiple sources, including a database covering about 10% of hospitalized patients in Germany. The results were shared with the public through an online dashboard that attracted up to 200,000 visits daily at its peak. The tool’s success led to its adoption in other countries, including Switzerland, Spain, Italy, France, Luxembourg, and the United States [1-3].
As part of the EUVABECO project, the University of Saarland is currently expanding the scope of its tool to work with any infectious disease. They are collaborating with partners in Greece, Luxembourg, Poland, and Portugal on pilot projects aimed at deploying a predictive model that incorporates data from infection and outcome studies, current infection rates of COVID-19 and other infections, including other respiratory diseases, as well as regional data on hospitalizations, Intensive Care Unit (ICU) admissions, and deaths. The model will also consider existing interventions, like vaccination campaigns, and specific local outbreak responses, including non-pharmaceutical interventions (NPIs).
Modelling tools like these provide important information that helps policymakers and healthcare providers make timely, informed decisions, allocate resources effectively, and implement life-saving interventions. These forecasts are vital for planning, allowing healthcare systems to prepare for future needs, such as ICU beds and specialized treatments. Additionally, these tools help educate the public on the importance of timely interventions and build trust in public health strategies.
The first draft of the implementation plans is currently in development and will be released to the public on the EUVABECO project website in October 2024, coinciding with the launch of the pilot projects. These plans will undergo rigorous testing and refinement during the pilot phase to ensure they provide comprehensive guidance – covering operational, technical, and legal aspects – for countries looking to establish their own modelling and forecasting tools. This will help countries to gain actionable insights during infectious disease outbreaks, strengthening their ability to respond to future public health emergencies.
References
[1] Nunes MC et al. Redefining pandemic preparedness: Multidisciplinary insights from the CERP modelling workshop in infectious diseases, workshop report. Infect Dis Model. 2024 Feb 23;9(2):501-518. doi: 10.1016/j.idm.2024.02.008. PMID: 38445252; PMCID: PMC10912817.
[2] Dings C et al. Model-Based Analysis of SARS-CoV-2 Infections, Hospitalization and Outcome in Germany, the Federal States and Districts. Viruses. 2022 Sep 24;14(10):2114. doi: 10.3390/v14102114. PMID: 36298669; PMCID: PMC9607468.
[3] Dings C et al. Effect of vaccinations and school restrictions on the spread of COVID-19 in different age groups in Germany. Infect Dis Model. 2024 Jul 24;9(4):1250-1264. doi: 10.1016/j.idm.2024.07.004. PMID: 39183948; PMCID: PMC11342094.